As Australian government issues guidance to workers on AI use, academic cites need for 'sufficient reliability'
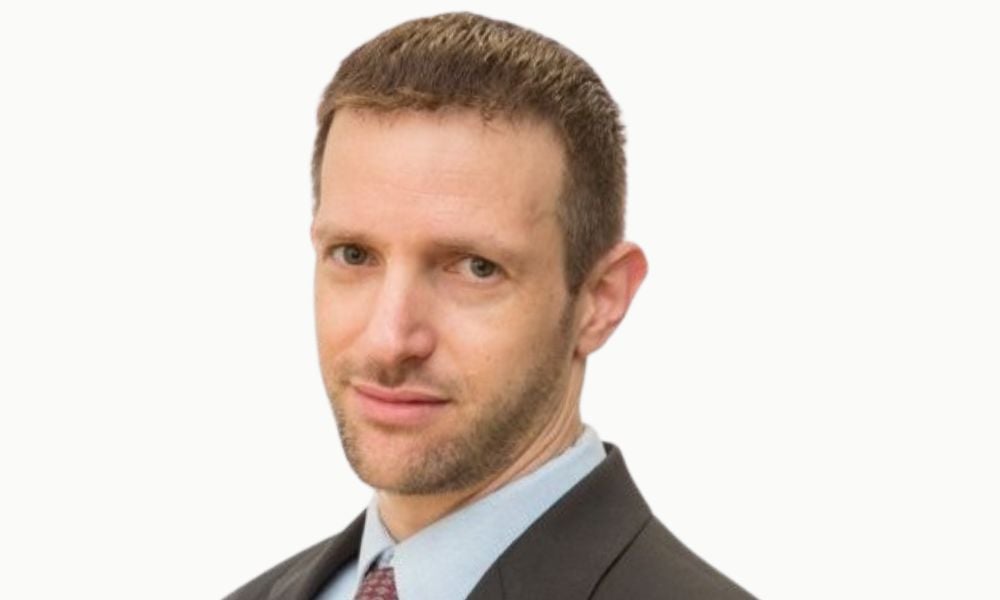
When the biggest employer in the country gets serious about training its workers in artificial intelligence (AI), you can be sure the wave is about to hit. The Australian government’s Digital Transformation Agency (DTA) has issued guidance on training in AI and policy for the responsible use of AI by government workers.
Public servants will be drilled on how AI works and when it is suitable to use generative AI, with agencies required to nominate accountable officials by 30 November.
“The policy strongly recommends that agencies implement AI fundamentals training for all staff, regardless of their role,” a statement from the DTA said.
An initiative on the scale of the DTA guidelines makes good sense, given the uncertainty and hype around AI, according to Uri Gal, a professor of business information systems at the University of Sydney Business School.
“Many people feel there’s a certain mysteriousness to this technology, and they’re not really sure what it is. There is so much hype that it can do anything – and better than humans can – and that maybe it is even conscious,” he said.
“The risk profile can be very significant, depending on the nature of the organisation or the industry.”
Understanding generative AI
Managers may be in the dark about the extent of AI’s powers, but they need to be responsible for implementing training programs, Gal said — and know how AI works.
“They first have to recognise the importance and the impact that this technology is likely to have, whether they take the initiative or not,” he said.
AI is “basically a very sophisticated statistical engine to generate output,” Gal said, meaning a probability function generates the next word in a sentence based on the likelihood it is the best word to add.
“There’s no brain or, I would argue, certainly no consciousness behind it,” he said.
Previous iterations of the technology were more deterministic, so that the same inputs returned the same output. Today’s large language models, such as ChatGPT, are probabilistic, so that the same input can return different results.
“There’s no way of knowing beforehand what’s going to get generated, so they are inherently unreliable,” Gal said. “That’s good for creative purposes, if you want to prompt the system to give you ideas, but if you want to use it to generate financial advice or a medical diagnosis, then reliability is your friend.”
Privacy, accuracy risks in using AI
Businesses that are working on AI training should zero in on privacy and risk, Gal said.
“You want to make sure you don’t compromise your employees’ or your customers’ privacy, as these systems work by dissecting huge amounts of data,” he said. “You want to make sure the data stays where it’s meant to be, and people who are not supposed to view it don’t have access to it.”
Next, there is the risk of AI generating wrong or misleading information, which may be let loose in a chatbot, for example.
“You want to make sure these tools are sufficiently reliable – they can never be entirely reliable – but sufficiently reliable so they don’t give wrong information that could lead to lawsuits or decreased reputation,” he said. “There are many cases where companies have suffered from this.”
And, of course, always follow the regulatory compliance regimes within your sector, Gal said: “Banking, medicine, healthcare – these are very crucial industries that need to keep a very tight lid on how they use these tools.”
AI usages for HR
The tech tool is increasingly being used for internal HR purposes, including performance management, hiring, promotion and firing. There are evident benefits, Gal said, in terms of saving time and resources in sifting thousands of resumes.
“But there’s a lot of research and examples of how AI tools can be biased, and they’re not designed to be biased,” he said. “They’re designed to eliminate bias, but it’s really hard to do so.”
For example, asked to ignore clues for ethnicity in a surname, AI might apply a bias correlated with race, such as postcode.
“It’s difficult to eliminate all these correlations that exist within a really large data set, and these data sets are massive,” Gal said.
AI has a tendency to deliver self-fulfilling prophecies; for example, winnowing applicants to retain the ones who are most like staff who have already reached the top.
“If you train the algorithm to identify the same traits applicants have that your current employees have, they keep hiring the same people – so there is less diversity in the workforce, and less innovation,” he said.
‘Take it with a grain of salt’
Despite the risks, Gal said he is not anti-AI.
He gives the example of organisations where workers have tested ways of prompting the tool to produce predictably reliable output, where the technology has saved time and led to higher-quality reports to clients.
“But that is one study, and I would take it with a grain of salt,” he said. “We don’t have sufficient, well-rounded, long-term evidence of what is the best collaboration [between humans and AI].”
It also depends on the task that AI is given. In banking and insurance, it has been used to detect fraud for decades, Gal said.
“But generative AI is a bit different, because it will generate outputs, and not just give you a static or descriptive recommendation.”