Incentivised workers will make smarter use of AI, find Kiwi, Aussie researchers
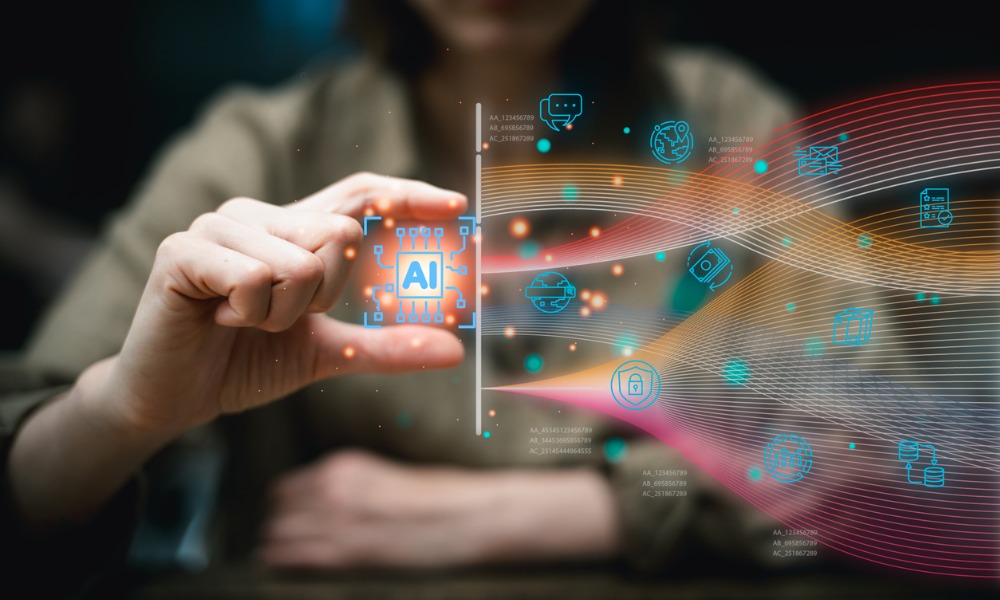
As businesses incorporate artificial intelligence (AI) into processes and services, many workers are increasingly worried.
In the United States, the sectors in which workers are most concerned about job security are those seen as most at risk of being impacted by AI, including software development (74%), finance and insurance (67%) and human resources (64%), according to research by marketing firm Authority Hacker.
But research out of New Zealand and Australia suggests these fears may be unfounded.
Research into artificial intelligence
In a bid to understand how workers might adapt to AI, Frank Ma, University of Auckland Business School lecturer, and Stijn Masschelein and Vincent Chong, University of Western Australia researchers, enlisted 161 people to participate in a series of online tasks.
The team wanted to gauge how AI would influence workers’ decision making. The experiment required participants to find a solution using output from an AI tool, or to override the AI tool and use their intuition, honed over iterations of the trial.
“In one condition, the participants can override or make their own decision from the very beginning,” Ma told HRD. “The other group only has the freedom to override the AI halfway through [the experiment], before which they must follow the decision made by the algorithm.”
The group that had the freedom to override AI from the very beginning learned much more than the group who had the freedom only to override AI in the second half.
Incentives help AI adoption
Performance was helped along by the possibility of incentives if a participant improved the algorithm’s decision by modifying it. If there was no chance of modifying the AI output in the first part of the experiment, there was nothing to learn.
Latest News
“When people could focus on the imperfectness of the algorithm, but couldn’t do anything about it, that bothered them and distracted from learning the main task,” Ma said.
Participants were asked to imagine they were a production manager whose job was to optimise output to meet market demand, where demand is unknown.
“If you over-produce, you lower the profit,” Ma said. “And if you under-produce, the profit will not be optimum.”
When an incentive is offered, a person’s motivation is to maximise their performance by setting production near optimal levels. This sees them focus on the imperfectness of the algorithm. That’s when learning kicks in, he said.
“They are then thinking about developing a strategy to find out the optimum demand in the market in the long run.”
Machine learning made human
Ma reached for a real-world example: “Say you are a financial analyst, for example, and your salary is hugely incentive-based. Sometimes you need to make a final prediction in addition to what is recommended in the system or by the AI,” he said.
“Or if you are a bank loan officer, sometimes you need to override the information in the decision-making tool because you know something that the system does not know. In a situation like that, you probably want to give people freedom from the very beginning.”
If a worker is not free to make decisions, they may become caught up in the imperfectness in the system, “and they cannot necessarily focus on performing their task,” Ma said.
With tasks that require significant cognitive skills, piece-rate incentive remuneration — along with the freedom to override the control system from the very beginning — will lead to a better result than tying workers’ hands and paying a flat wage, found the researchers.